Key points
This is a regular/credit-earning course for Statistics PhD students at North Carolina State University. The lectures take place biweekly (Tuesdays & Thursdays, 11:45am–1:00pm, in SAS Hall 5270) — starting on Tuesday, August 23rd, ending in early December — and the video recordings, slides, etc. will be posted on the Course Materials page after each lecture.
Grades for the registered students will be based on the following assessments:
- reading, occasional homework, and general “class participation”
- a final course project.
Some more details about these assessments (especially the project) are given below, with additional clarity to be provided as the course progresses.
An “official” course syllabus can be downloaded here. Registered students can access the Moodle page to view the grade book.
Office hour schedule:
- Wednesday 3–4pm on Zoom (updated 09/14, link on the Moodle page)
- Thursday 1–2pm in person (my office)
Further details
Instructor information
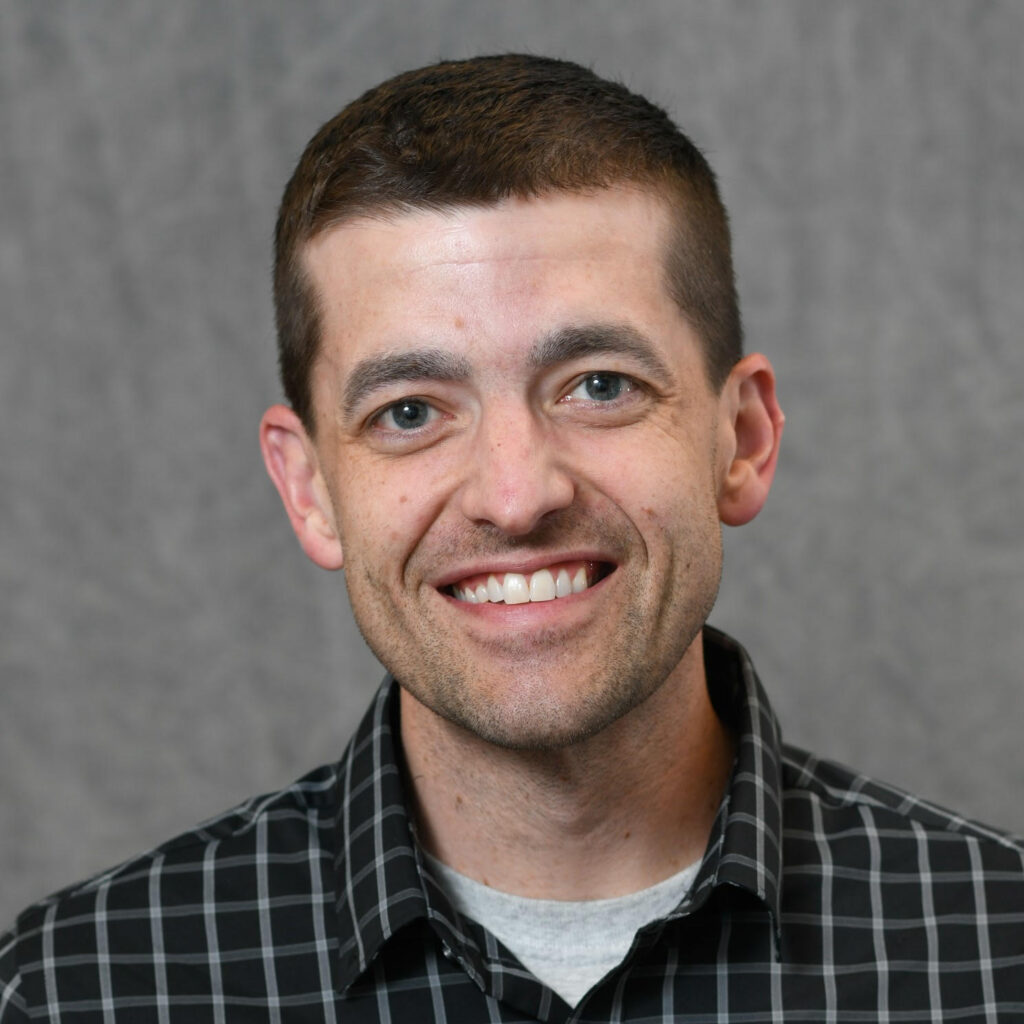
- Dr. Ryan Martin
- Email: rgmarti3@ncsu.edu
- Professor of Statistics, North Carolina State University
- PhD in Statistics, Purdue University, 2009
- Co-founder of Researchers.One
- Co-author of Inferential Models
- Research broadly focuses on the foundations of statistics and imprecise probability; working and published papers
Prerequisites/assumed background
Background in probability and mathematical statistics as covered in Casella & Berger’s Statistical Inference text (e.g., ST701–702 at NC State) is expected. Familiarity with advanced probability (e.g., ST779 at NC State), advanced statistical inference (e.g., ST793 at NC State), and Bayesian inference (e.g., ST540/740 at NC State) would be beneficial but isn’t necessary.
The instructor will do computations using the R statistical software package, which is freely available to download and install from CRAN, but students are welcome to use whatever software they like.
Rough course outline
The course will roughly be split into two parts.
Part 1 will focus on the various imprecise probability models themselves, both on intuition/motivation and technical details & properties. I’ll focus mainly on possibility measures, random sets, belief functions, and lower/upper previsions, but a few other models may pop up as time allows. Relevant book references are listed under the Resources tab.
Part 2 will explore some applications of imprecise probability in statistics and machine learning. The specific topics/applications to be covered have yet to be determined but, for sure, this will be a biased selection. In particular, some time will definitely be spent covering the instructor’s recent developments on possibility theory, inferential models, and the foundations of statistical inference.
The course project, discussed below, is where the students will have an opportunity to tailor their course experience more towards their own interests…
Course project
More details will be provided as the course progresses, here is just a big-picture description of what the instructor has in mind.
Despite the substantial developments in both imprecise probability and statistics & machine learning, the intersection of the two is ripe for exploration. So, an example of a course project is one where students (individually or in pairs) identify an application area of interest to them, say, causal inference, do a review of the relevant literature (both stat/ML & IP), and formulate in writing some ideas on how something new might be done. The instructor is happy to offer some suggestions and guidance to help the students along the way. It’s not out of the question that these course projects could evolve into journal/conference papers (e.g., for the upcoming ISIPTA’23) or more substantial, long-term investigations.